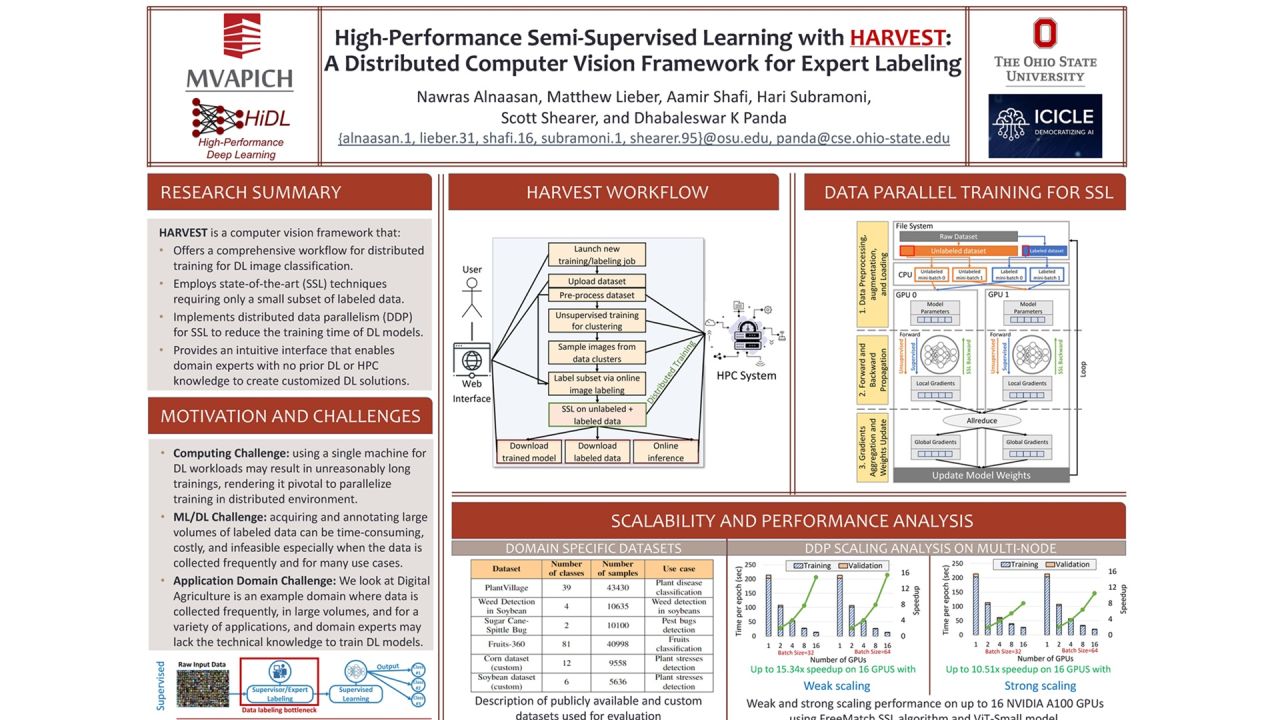
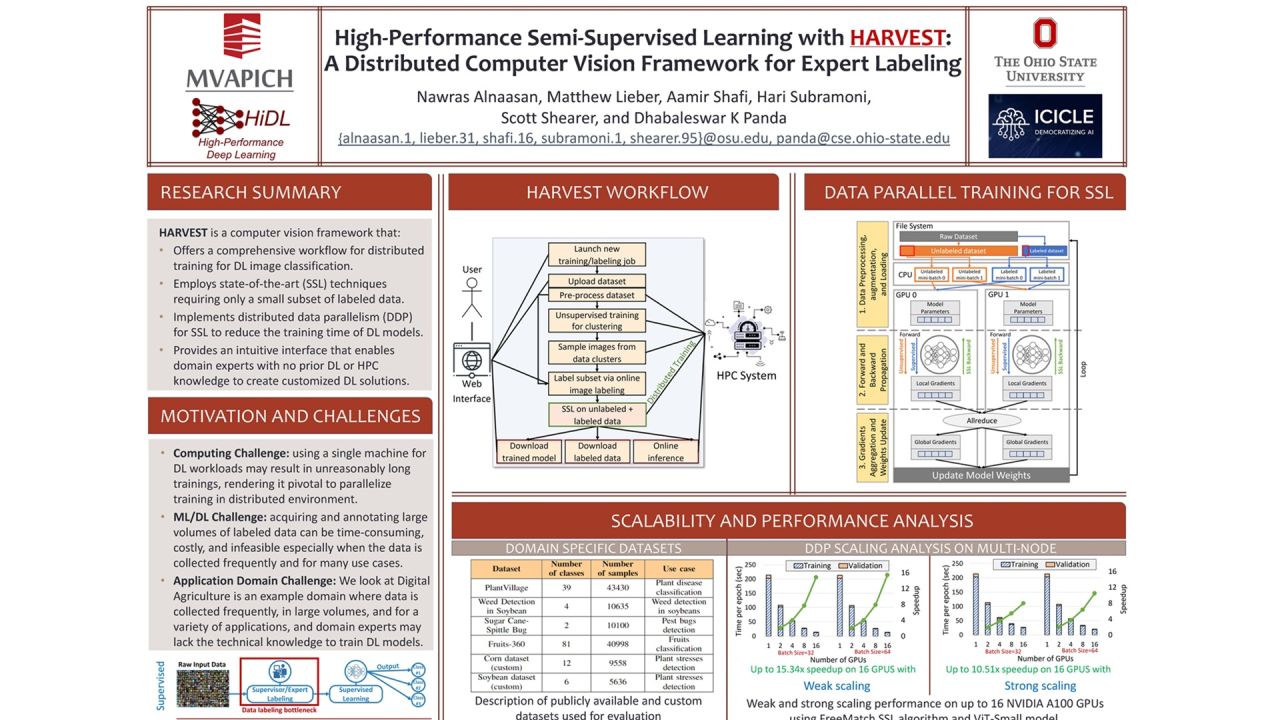
High-Performance Semi-Supervised Learning with HARVEST: A Distributed Computer Vision Framework for Expert Labeling (Best Poster Finalist)
Monday, May 13, 2024 3:00 PM to Wednesday, May 15, 2024 4:00 PM · 2 days 1 hr. (Europe/Berlin)
Foyer D-G - 2nd floor
Research Poster
AI Applications powered by HPC TechnologiesHPC System Design for Scalable Machine LearningML Systems and ToolsScalable Application Frameworks
Information
Poster is on display and will be presented at the poster pitch session.
Supervised Deep Learning (DL) thrives on data; however, it inherits a major limitation—training and testing datasets must be fully annotated to train Deep Neural Networks (DNNs). To mitigate this bottleneck, we propose HARVEST—a distributed computer-vision framework that employs state-of-the-art semi-supervised learning (SSL) algorithms to train robust DNNs using Distributed Data Parallelism (DDP) on High-Performance Computing (HPC) systems with only a small subset of labeled data samples. HARVEST offers an intuitive and interactive web-based interface that enables domain experts with no prior DL or HPC knowledge to easily unlock the power of DL and leverage the computational resources offered by HPC systems, furthering the mission of democratizing AI. We conduct a comprehensive evaluation of several domain-specific use cases as that can benefit from HARVEST where data is collected frequently, in large volumes, and for a variety of applications. Our evaluations yield accuracies within 3% compared to fully supervised training using less than 80 labeled samples per class. Furthermore, we show that HARVEST delivers near-linear scaling, reducing the training time from 7.8 hours on a single NVIDIA A100 GPU up to 31 minutes by using DDP on 16 GPUs. To the best of our knowledge, HARVEST is the first framework that allows end-users to perform interactive labeling and distributed training using state-of-the-art SSL algorithms.
Contributors:
Supervised Deep Learning (DL) thrives on data; however, it inherits a major limitation—training and testing datasets must be fully annotated to train Deep Neural Networks (DNNs). To mitigate this bottleneck, we propose HARVEST—a distributed computer-vision framework that employs state-of-the-art semi-supervised learning (SSL) algorithms to train robust DNNs using Distributed Data Parallelism (DDP) on High-Performance Computing (HPC) systems with only a small subset of labeled data samples. HARVEST offers an intuitive and interactive web-based interface that enables domain experts with no prior DL or HPC knowledge to easily unlock the power of DL and leverage the computational resources offered by HPC systems, furthering the mission of democratizing AI. We conduct a comprehensive evaluation of several domain-specific use cases as that can benefit from HARVEST where data is collected frequently, in large volumes, and for a variety of applications. Our evaluations yield accuracies within 3% compared to fully supervised training using less than 80 labeled samples per class. Furthermore, we show that HARVEST delivers near-linear scaling, reducing the training time from 7.8 hours on a single NVIDIA A100 GPU up to 31 minutes by using DDP on 16 GPUs. To the best of our knowledge, HARVEST is the first framework that allows end-users to perform interactive labeling and distributed training using state-of-the-art SSL algorithms.
Contributors:
Format
On-site