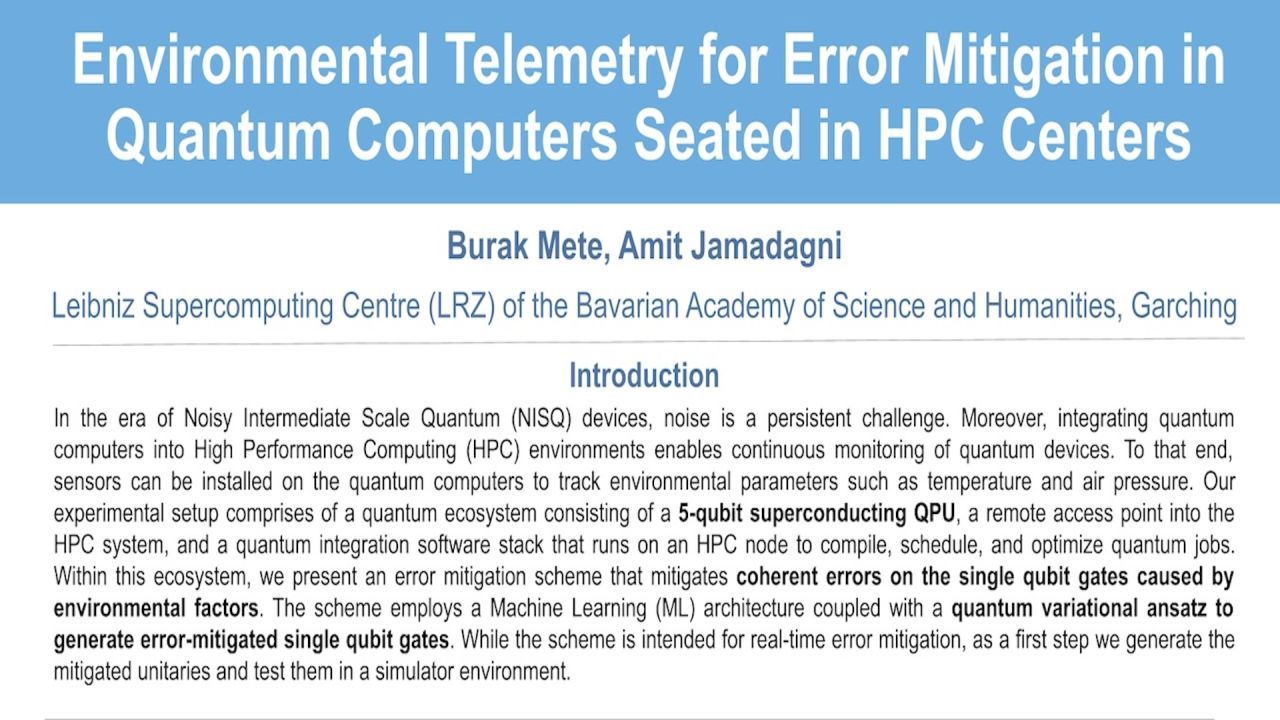
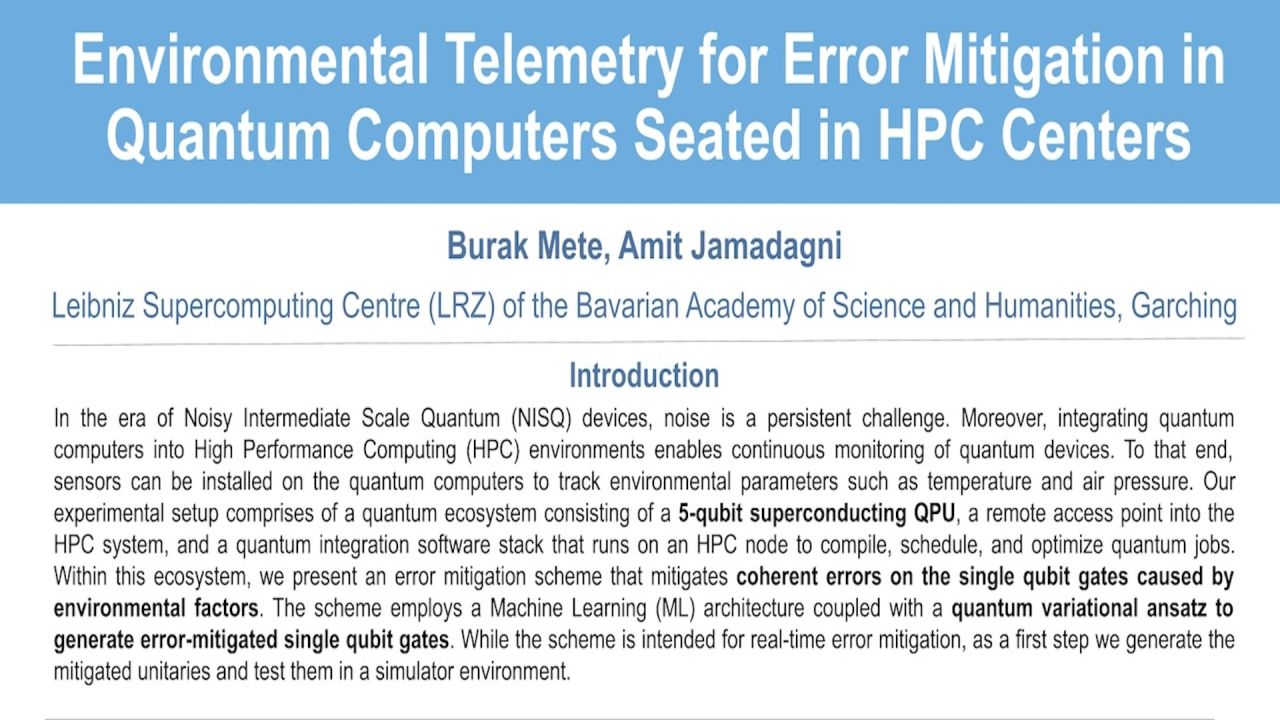
Environmental Telemetry for Error Mitigation in Quantum Computers Seated in HPC Centers
Monday, May 13, 2024 3:00 PM to Wednesday, May 15, 2024 4:00 PM · 2 days 1 hr. (Europe/Berlin)
Foyer D-G - 2nd floor
Research Poster
Integration of Quantum Computing and HPCML Systems and ToolsQuantum Computing - Basics and TheoryQuantum Computing - Technologies and ArchitecturesQuantum-inspired Algorithms and Technologies
Information
Poster is on display and will be presented at the poster pitch session.
High Performance Computing (HPC) centers have seasoned and expansive experience monitoring supercomputing architectures for operations and performance tracking. This continuous monitoring is enabled by their ability to store and process large databases for event response, usage tracking and strategic decision making. As various quantum computing (QC) architectures mature and their dependence on HPC compute for various use cases increases, the develop of hybrid workflows emerges. Additionally, the importance of integrating QC hardware into HPC and data centers and their telemetry infrastructure becomes apparent. The current generation of quantum devices must account for excessive calculation errors and instability, referred to as the Noisy Intermediate Scale Quantum (NISQ) era, with various noise sources arising from fabrication limitations and from the external surroundings. To track the latter, we equip the environment of QC hardware with sensors that record several environmental fluctuations and further present a Machine Learning (ML) framework integrated with variational quantum circuits that map the same to a unitary. We employ a supervised learning strategy and train the neural network on the sensor data with the output being parameters of a variational quantum ansatz that represents the noisy unitary. The loss function employed relates the expectation value obtained from numerical simulation with that of the experimental data. To demonstrate the method, we monitor the environment of a QC based on superconducting qubits and further estimate the noise from the environment.
Contributors:
High Performance Computing (HPC) centers have seasoned and expansive experience monitoring supercomputing architectures for operations and performance tracking. This continuous monitoring is enabled by their ability to store and process large databases for event response, usage tracking and strategic decision making. As various quantum computing (QC) architectures mature and their dependence on HPC compute for various use cases increases, the develop of hybrid workflows emerges. Additionally, the importance of integrating QC hardware into HPC and data centers and their telemetry infrastructure becomes apparent. The current generation of quantum devices must account for excessive calculation errors and instability, referred to as the Noisy Intermediate Scale Quantum (NISQ) era, with various noise sources arising from fabrication limitations and from the external surroundings. To track the latter, we equip the environment of QC hardware with sensors that record several environmental fluctuations and further present a Machine Learning (ML) framework integrated with variational quantum circuits that map the same to a unitary. We employ a supervised learning strategy and train the neural network on the sensor data with the output being parameters of a variational quantum ansatz that represents the noisy unitary. The loss function employed relates the expectation value obtained from numerical simulation with that of the experimental data. To demonstrate the method, we monitor the environment of a QC based on superconducting qubits and further estimate the noise from the environment.
Contributors:
Format
On-site