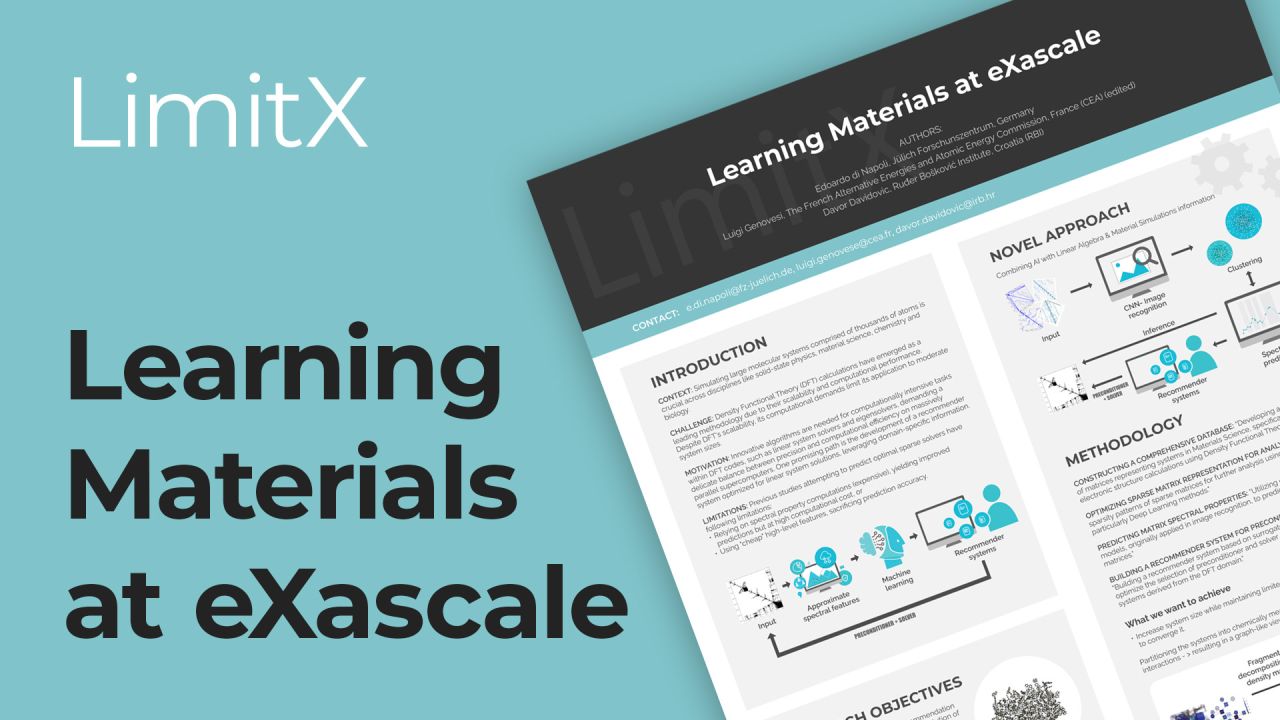
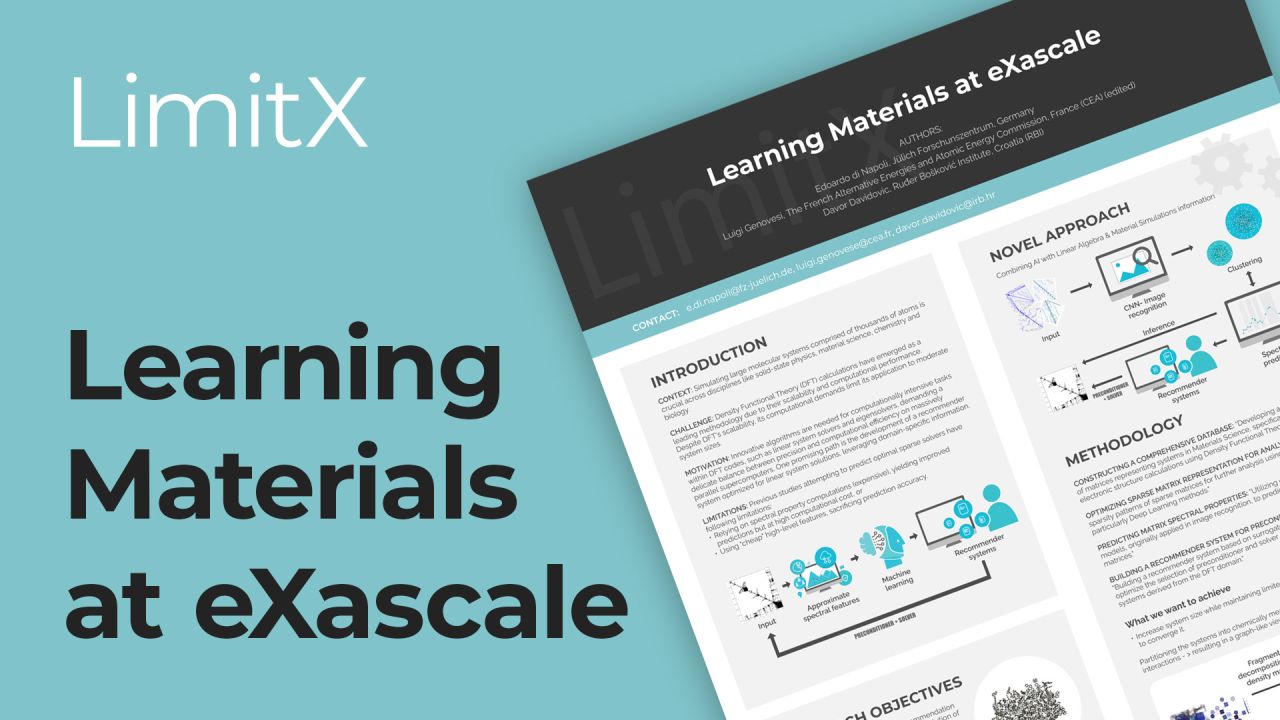
Learning Materials at eXascale (LimitX)
Monday, May 13, 2024 3:00 PM to Wednesday, May 15, 2024 4:00 PM · 2 days 1 hr. (Europe/Berlin)
Foyer D-G - 2nd floor
Project Poster
Extreme-scale AlgorithmsHPC Simulations enhanced by Machine LearningNovel AlgorithmsNumerical Libraries
Information
Poster is on display.
Solving large and sparse numerical linear systems in materials science on massively parallel supercomputers presents a formidable challenge and requires a delicate balance between precision and computational efficiency. The LimitX project represents a pioneering effort to redefine the resolution of large-scale sparse linear systems by accelerating and scaling their solutions. The core of this system consists of a two-pronged approach: first, the development of a spectral predictor system, and second, the use of an extensive matrix database that encompasses the fundamental characteristics of surrogate spaces in materials science. The spectral predictor system is the heart of the recommendation system. Using advanced deep learning techniques, it predicts spectral properties critical for the efficient solution of linear systems. At the same time, the comprehensive matrix dataset captures the diverse spectral patterns that occur in material science calculations. The application of this recommender system promises to be groundbreaking as it enables simulations with hundreds of thousands of atoms, a feat previously unattainable on existing pre-exascale clusters. The linear scaling of Density Functional Theory (DFT) codes such as BigDFT will enable researchers to simulate and analyse complex material systems with unprecedented accuracy and computational efficiency, opening up new horizons for scientific exploration in materials science.
Contributors:
Solving large and sparse numerical linear systems in materials science on massively parallel supercomputers presents a formidable challenge and requires a delicate balance between precision and computational efficiency. The LimitX project represents a pioneering effort to redefine the resolution of large-scale sparse linear systems by accelerating and scaling their solutions. The core of this system consists of a two-pronged approach: first, the development of a spectral predictor system, and second, the use of an extensive matrix database that encompasses the fundamental characteristics of surrogate spaces in materials science. The spectral predictor system is the heart of the recommendation system. Using advanced deep learning techniques, it predicts spectral properties critical for the efficient solution of linear systems. At the same time, the comprehensive matrix dataset captures the diverse spectral patterns that occur in material science calculations. The application of this recommender system promises to be groundbreaking as it enables simulations with hundreds of thousands of atoms, a feat previously unattainable on existing pre-exascale clusters. The linear scaling of Density Functional Theory (DFT) codes such as BigDFT will enable researchers to simulate and analyse complex material systems with unprecedented accuracy and computational efficiency, opening up new horizons for scientific exploration in materials science.
Contributors:
Format
On-site
Registered attendees
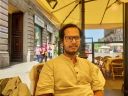
Abhiram Kaushik Badrinarayanan
Postdoctoral researcherRudjer Boskovic Institute