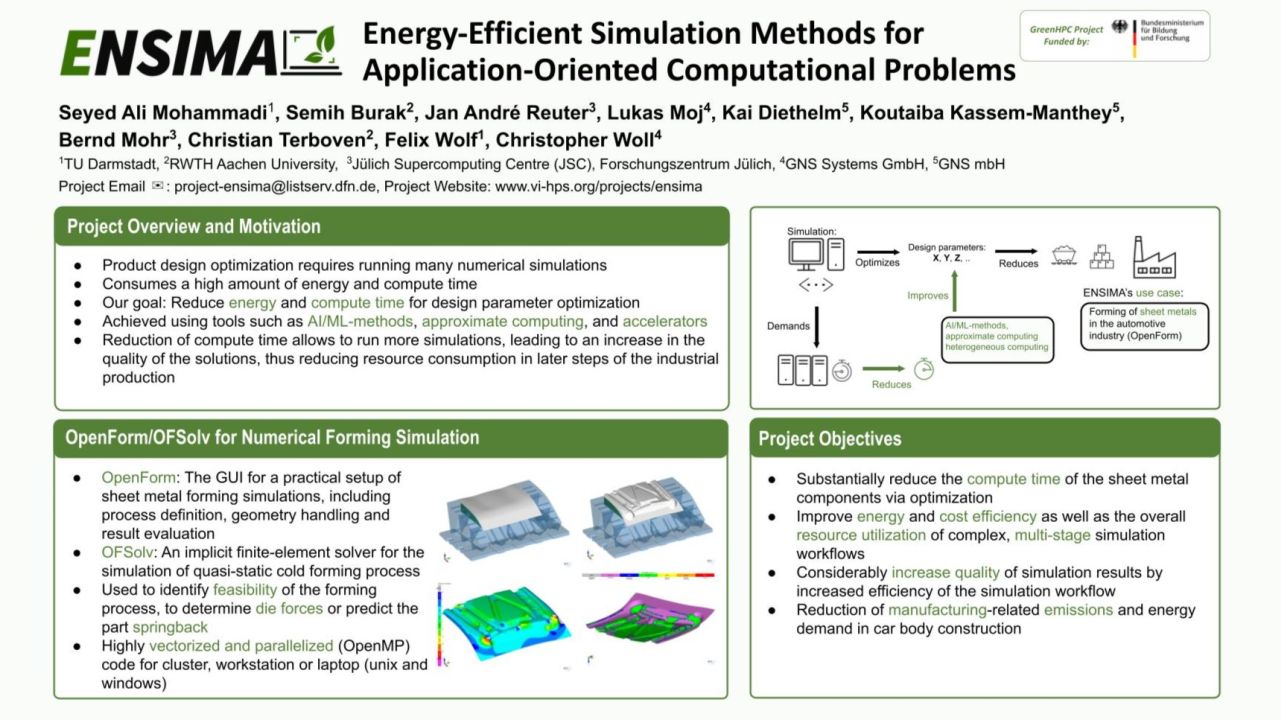
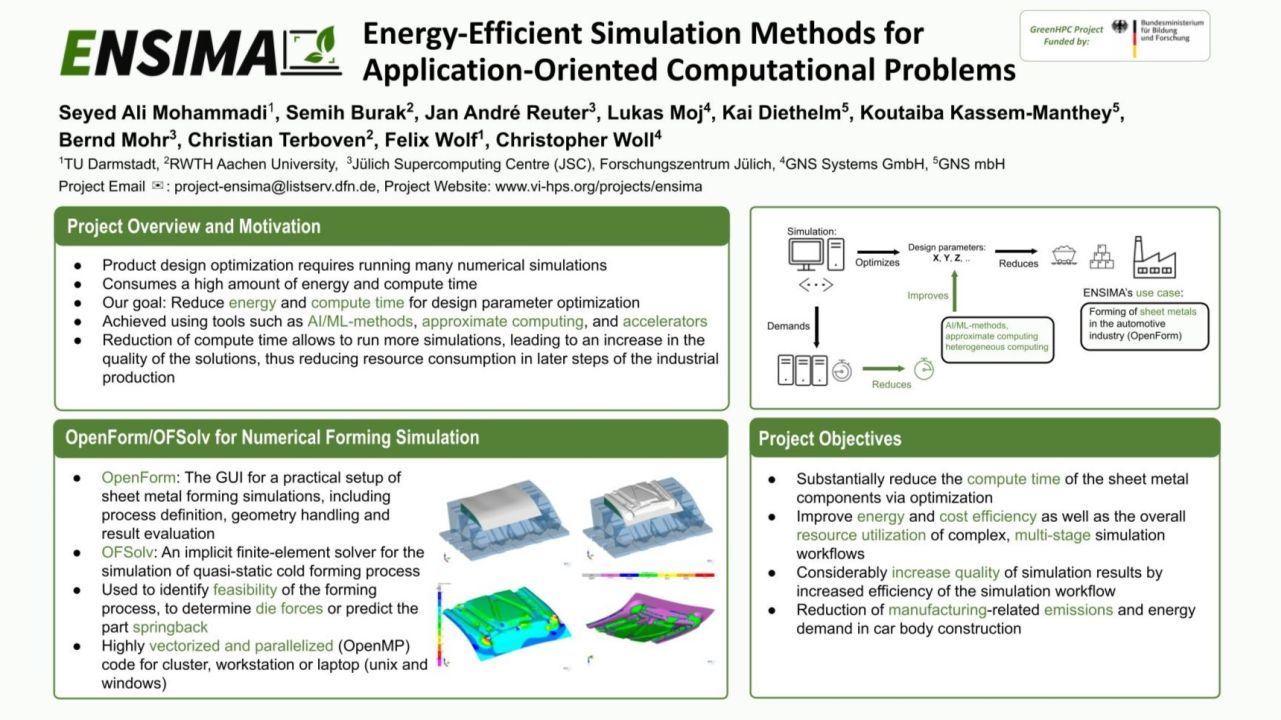
ENSIMA: Energy-Efficient Simulation Methods for Application-Oriented Computational Problems
Monday, May 13, 2024 3:00 PM to Wednesday, May 15, 2024 4:00 PM · 2 days 1 hr. (Europe/Berlin)
Foyer D-G - 2nd floor
Project Poster
HPC Simulations enhanced by Machine LearningIndustrial Use Cases of HPC, ML and QCOptimizing for Energy and PerformancePerformance and Resource ModelingSystem and Performance Monitoring
Information
Poster is on display.
Modern computer simulation capabilities can transform the industrial product design process by optimizing many design parameters while saving resources in production. However, in-process optimization often requires enormous computing time on HPC systems. In the ENSIMA project, the determination of design parameters is improved and accelerated by AI methods. To this end, a workflow for the design process has been proposed, which consists of a deep neural network that considers the properties of the material, the process parameters, and representative geometry parameters to predict variable process parameters; these predictions are later used as the initial configurations for the optimization process. For ENSIMA's use case – sheet metal forming in the automotive industry via the software OpenForm – the goal is to reduce computing time and steel usage to decrease manufacturing-related emissions and energy requirements indirectly. As part of ENSIMA, the support for Score-P measurement infrastructure will be expanded to include OpenMP offload and Intel's Level Zero. ENSIMA also improves the energy and cost efficiency and the overall resource utilization. Initial results on measuring the energy consumption show that for a fixed problem, the energy consumption diverges when certain design parameters are varied. This opens up the potential to extend the workflow for the sample selection by considering energy predictions, i.e., cost-aware sample selection. The project's next steps include finalizing the workflow and AI models, preparing a well-scaling application to utilize accelerators, applying approximate computing, evaluating the total cost of ownership, and transferring the methods to injection molding and cloud computing.
Contributors:
Modern computer simulation capabilities can transform the industrial product design process by optimizing many design parameters while saving resources in production. However, in-process optimization often requires enormous computing time on HPC systems. In the ENSIMA project, the determination of design parameters is improved and accelerated by AI methods. To this end, a workflow for the design process has been proposed, which consists of a deep neural network that considers the properties of the material, the process parameters, and representative geometry parameters to predict variable process parameters; these predictions are later used as the initial configurations for the optimization process. For ENSIMA's use case – sheet metal forming in the automotive industry via the software OpenForm – the goal is to reduce computing time and steel usage to decrease manufacturing-related emissions and energy requirements indirectly. As part of ENSIMA, the support for Score-P measurement infrastructure will be expanded to include OpenMP offload and Intel's Level Zero. ENSIMA also improves the energy and cost efficiency and the overall resource utilization. Initial results on measuring the energy consumption show that for a fixed problem, the energy consumption diverges when certain design parameters are varied. This opens up the potential to extend the workflow for the sample selection by considering energy predictions, i.e., cost-aware sample selection. The project's next steps include finalizing the workflow and AI models, preparing a well-scaling application to utilize accelerators, applying approximate computing, evaluating the total cost of ownership, and transferring the methods to injection molding and cloud computing.
Contributors:
Format
On-site