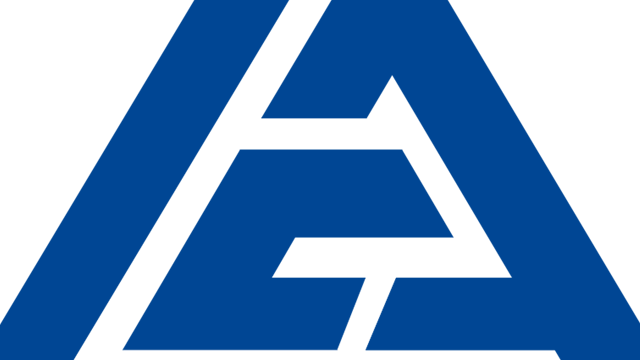
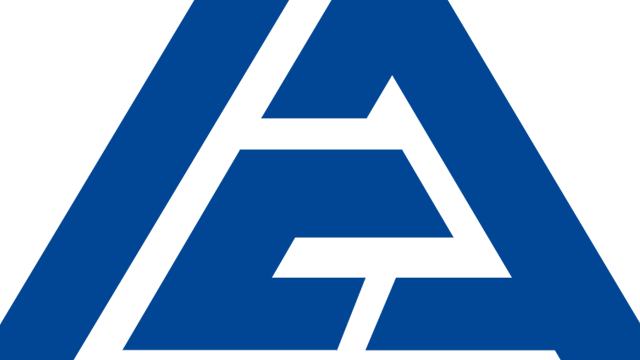
High-Performance Computing on Edge TPU: Performance Benchmarking of Real-Time Inferencing of Deep Learning Human Pose Estimation Models
Monday, May 13, 2024 3:00 PM to Wednesday, May 15, 2024 4:00 PM · 2 days 1 hr. (Europe/Berlin)
Foyer D-G - 2nd floor
Women in HPC
AI Applications powered by HPC TechnologiesEmerging Computing TechnologiesIndustrial Use Cases of HPC, ML and QCPerformance Measurement
Information
Poster is on display and will be presented at the poster pitch session.
This research explores the intersection of robotics, edge artificial intelligence (AI), and high-performance computing (HPC) with a focus on accelerating inference tasks using Tensor Processing Units (TPUs). The proliferation of edge computing in robotics demands efficient AI solutions for real-time decision-making within resource-constrained environments. In this study, we present a novel approach to harness the computational power of TPUs for enhancing the inference capabilities of robotic systems at the edge. Our methodology enables accelerated processing of deep learning-based human pose estimation models on TPUs with the Robot Operating System (ROS). We benchmarked the performance of popular pose recognition models - PoseNet -ResNet50, PoseNet-MobileNet V1, MoveNet Lightning and MoveNet Thunder. We also investigated the impact of TPU-accelerated inference on the overall performance of robotic systems compared to inferencing on CPU, considering factors such as inferencing time and accuracy. The results show that on TPU, MoveNet Lightning was the fastest, with 48 ms with a pose score of 0.98, and PoseNet-ResNet50 was the slowest among all the models, with 701 ms and a pose score of 0.93 .
This research explores the intersection of robotics, edge artificial intelligence (AI), and high-performance computing (HPC) with a focus on accelerating inference tasks using Tensor Processing Units (TPUs). The proliferation of edge computing in robotics demands efficient AI solutions for real-time decision-making within resource-constrained environments. In this study, we present a novel approach to harness the computational power of TPUs for enhancing the inference capabilities of robotic systems at the edge. Our methodology enables accelerated processing of deep learning-based human pose estimation models on TPUs with the Robot Operating System (ROS). We benchmarked the performance of popular pose recognition models - PoseNet -ResNet50, PoseNet-MobileNet V1, MoveNet Lightning and MoveNet Thunder. We also investigated the impact of TPU-accelerated inference on the overall performance of robotic systems compared to inferencing on CPU, considering factors such as inferencing time and accuracy. The results show that on TPU, MoveNet Lightning was the fastest, with 48 ms with a pose score of 0.98, and PoseNet-ResNet50 was the slowest among all the models, with 701 ms and a pose score of 0.93 .
Format
On-site