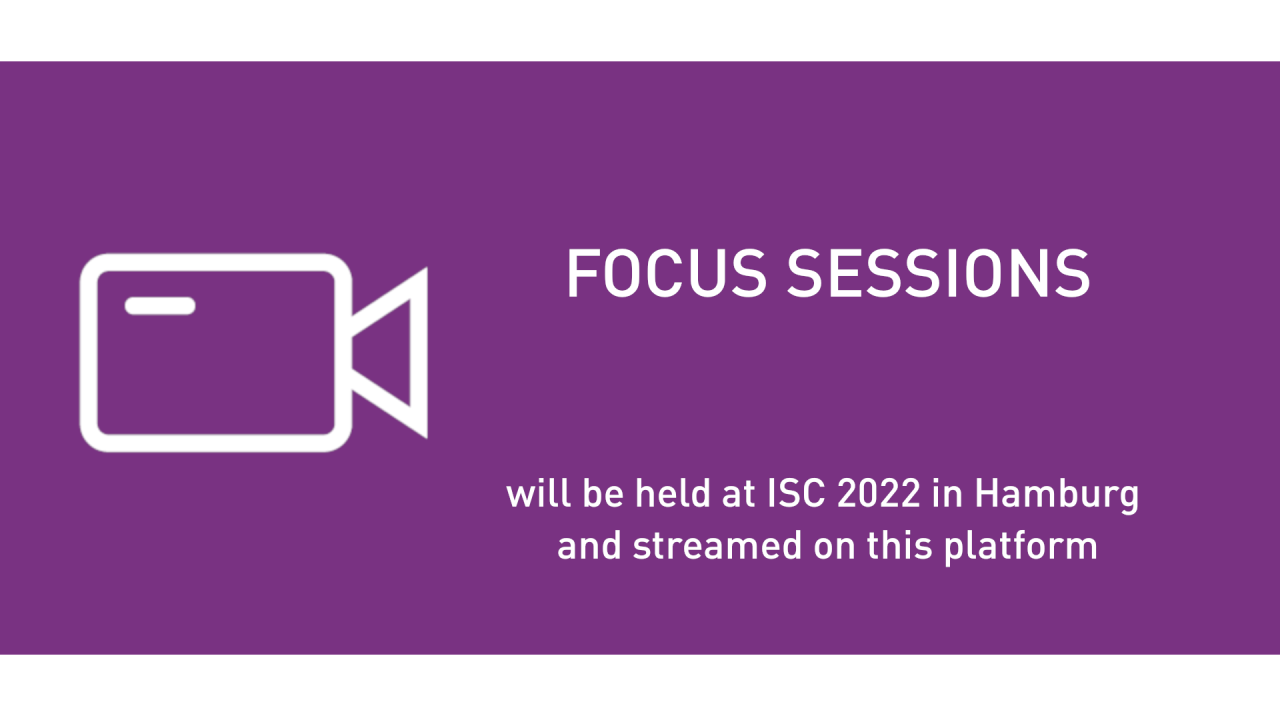
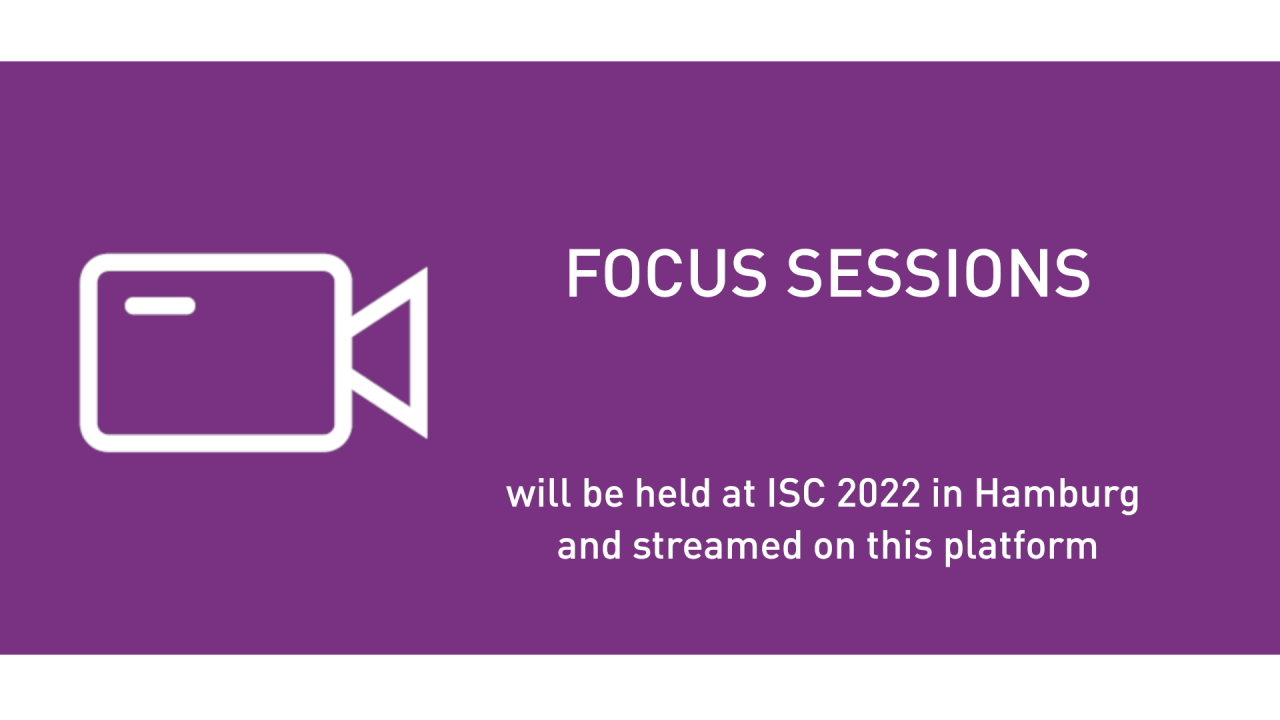
On the use of machine learning for subseasonal-to-seasonal predictions
Wednesday, June 1, 2022 2:15 PM to 2:35 PM · 20 min. (Europe/Berlin)
Hall 4 - Ground Floor
Information
Subseasonal-to-seasonal (S2S) predictions cover the gap between weather forecasting and climate projections, generating probabilistic forecasts up to six months ahead. The feasibility of S2S predictions largely rests on slow and predictable variations in soil moisture, snow cover, sea-ice, and ocean surface temperature, and how the atmosphere interacts and is affected by these boundary conditions. Probabilistic information from S2S forecasts has proven valuable for a wide range of societal sectors such as health, agriculture, or the renewable energy sector, boosting the research community's interest in making such predictions more skilful and reliable. Existing approaches strongly rely on General Circulation Models (GCMs), which suffer from systematic biases and drifts and present coarser horizontal spatial resolutions than those needed for regional or local applications. Machine learning (ML) models are universal approximators that represent highly complex nonlinear Spatio-temporal and multi-variable relationships while being capable of blending inputs from different data sources even with diverse formats and structures. Thus, ML models are potential candidates for overcoming the existing limitations of S2S predictions. In this talk, we review various opportunities presented by ML for S2S applications, together with their main practical challenges. We showcase a few selected ML implementations that aim to improve the predictive skill and resolution of state of the art GCMs. The first use case applies Convolution Neural Networks (CNNs) to upscale the spatial resolution of the ECMWF's seasonal prediction system (SEAS5), improving its spatial resolution (~111 km) by a factor of 10. The second use case blends an ensemble of ML methods to calibrate the ECMWF extended range forecast of temperature and precipitation targeting the forthcoming 3 to 6 weeks. This approach ranked second in the World Meteorological Organization's (WMO) S2S-AI challenge.
Format
On-siteLive-Online