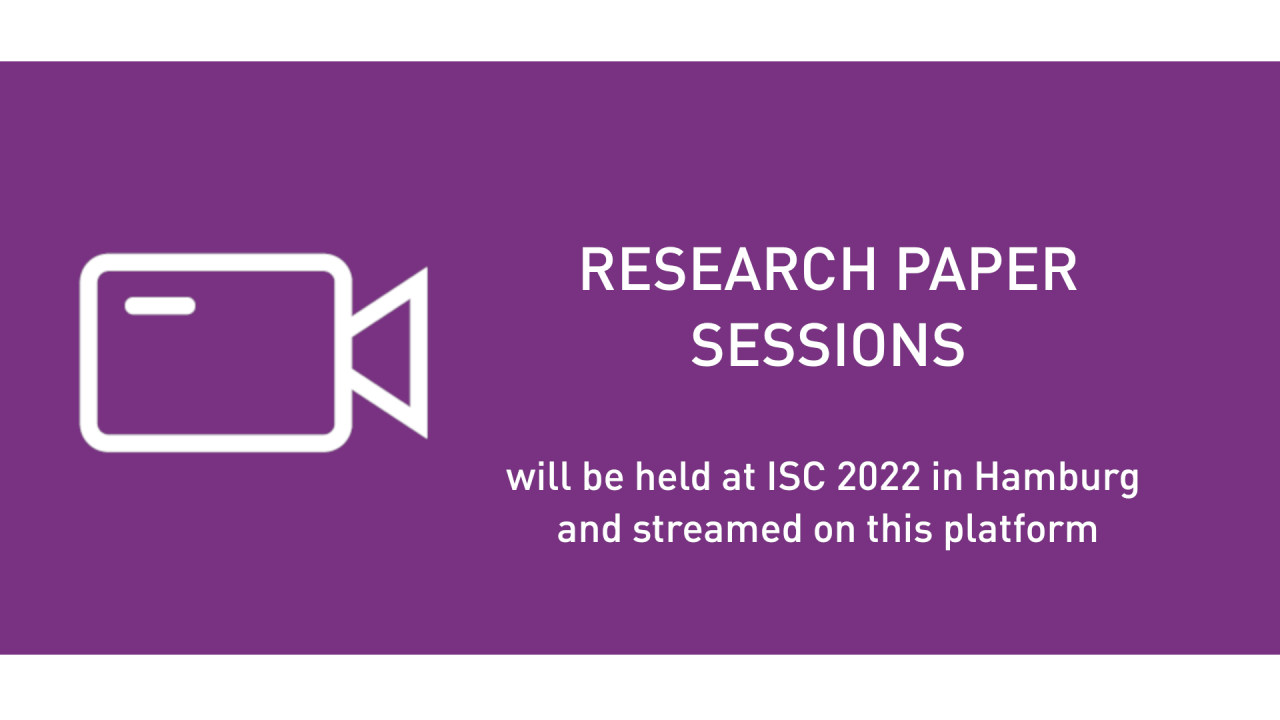
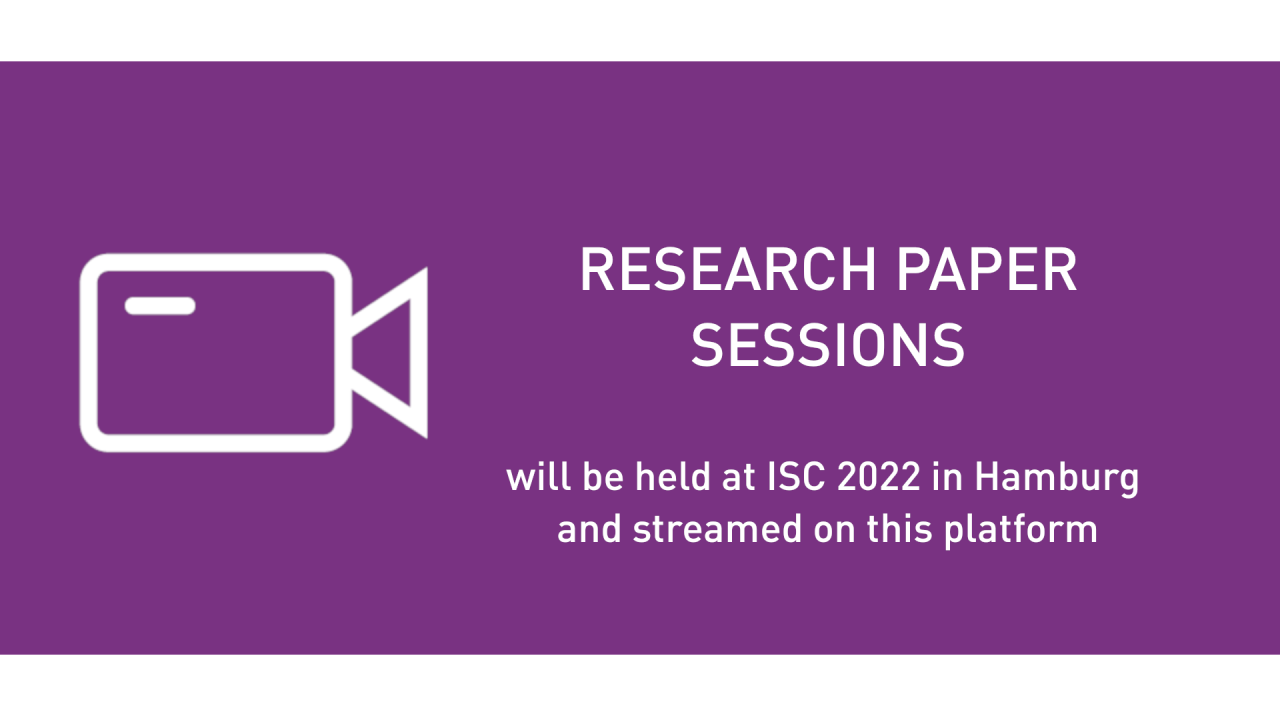
Rapid Execution Time Estimation for Heterogeneous Memory Systems through Differential Tracing
Tuesday, May 31, 2022 9:20 AM to 9:40 AM · 20 min. (Europe/Berlin)
Hall G1 - 2nd Floor
Information
As compute nodes complexity in high-performance computing (HPC) keeps increasing, systems equipped with heterogeneous memory devices are becoming paramount. Efficiently utilizing heterogeneous memory based systems, however, poses significant challenges to application developers. System software level transparent solutions utilizing artificial intelligence and machine learning based approaches, in particular non-supervised learning based methods such as reinforcement learning, may come to the rescue. However, such methods require rapid estimation of execution runtime as a function of the data layout across memory devices for exploring different data placement strategies, rendering architecture-level simulators impractical for this purpose.
In this paper, we propose a differential tracing based approach using memory access traces obtained by high-frequency sampling-based methods (e.g., Intel's PEBS) on real hardware running out of different memory devices. We develop a runtime estimator based on such traces that provides an execution time estimate orders of magnitudes faster than full system simulators. On a number of HPC mini-applications we show that the estimator predicts runtime with an average error of 4.4% compared to measurements on real hardware.
Contributors:
In this paper, we propose a differential tracing based approach using memory access traces obtained by high-frequency sampling-based methods (e.g., Intel's PEBS) on real hardware running out of different memory devices. We develop a runtime estimator based on such traces that provides an execution time estimate orders of magnitudes faster than full system simulators. On a number of HPC mini-applications we show that the estimator predicts runtime with an average error of 4.4% compared to measurements on real hardware.
Contributors:
- Nicolas Denoyelle (Argonne National Laboratory)
- Balazs Gerofi (RIKEN Center for Computational Science)
- Kamil Iskra (Argonne National Laboratory)
- Swann Perarnau (Argonne National Laboratory)
Format
On-siteLive-Online