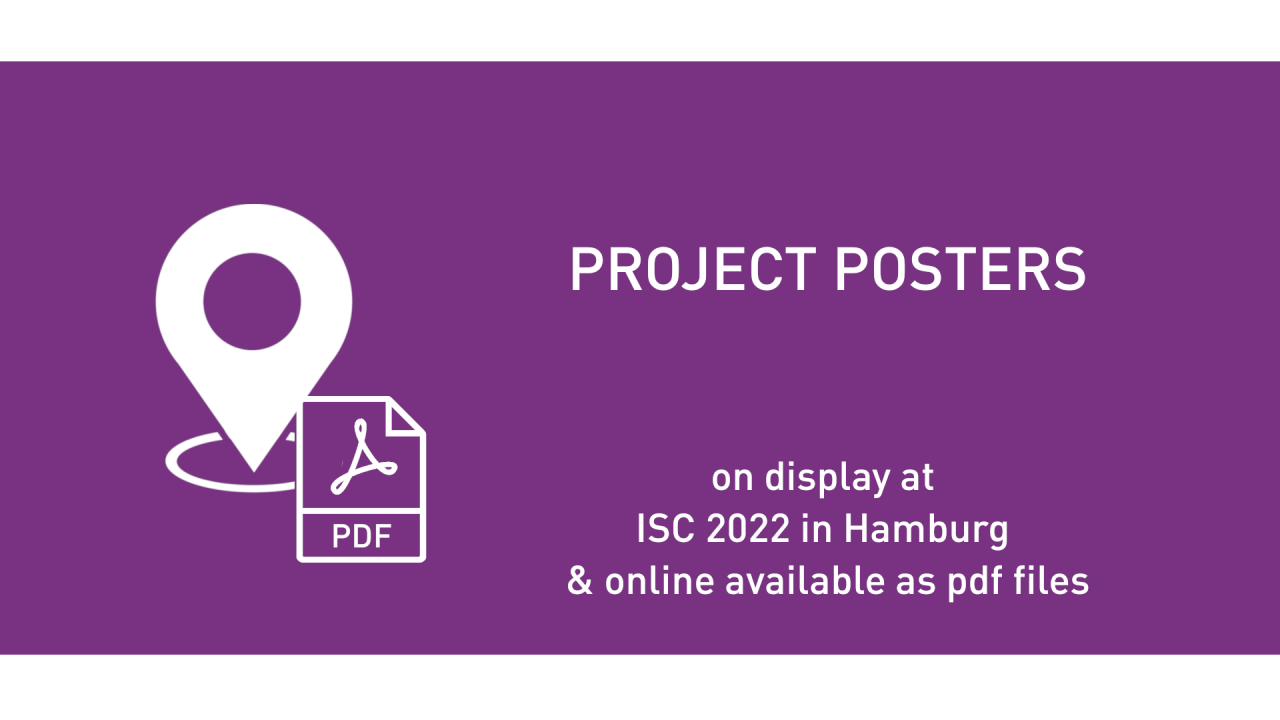
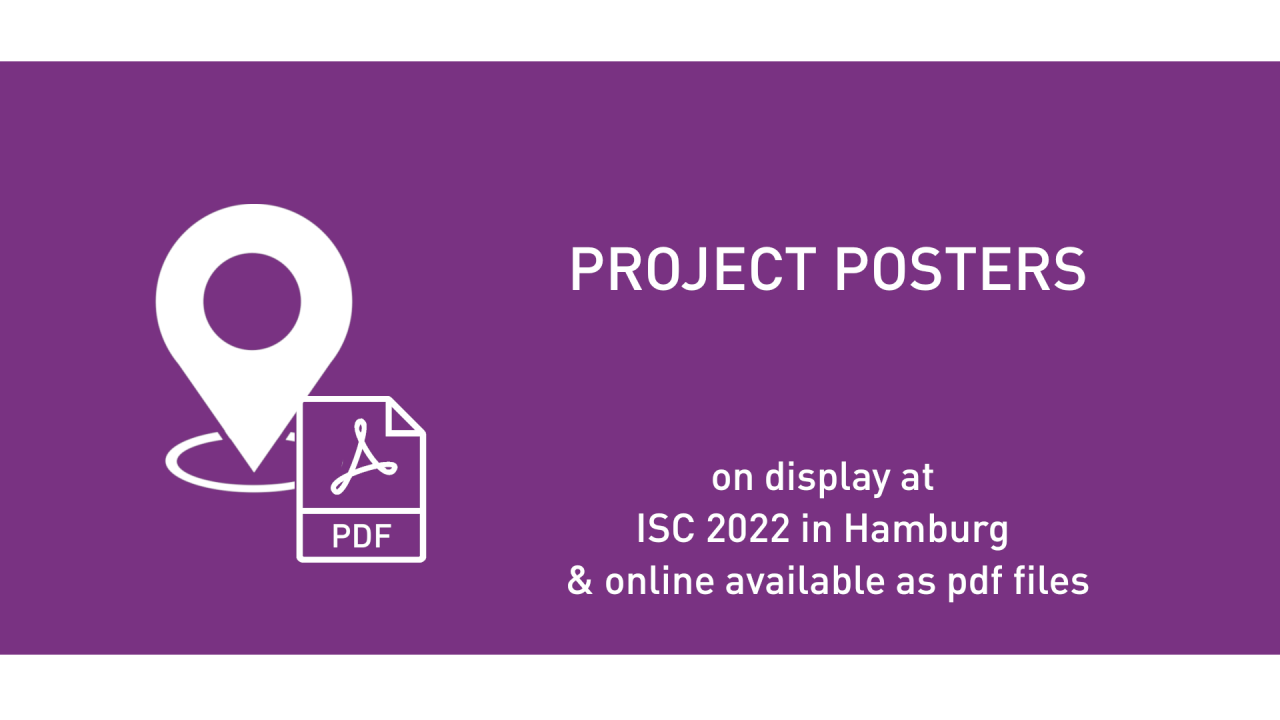
Visualize, Monitor and Control the Training Process of a Deep Surrogate Model in ParaView
Tuesday, May 31, 2022 9:00 AM to 6:30 PM · 9 hr. 30 min. (Europe/Berlin)
Foyer 3 + H - Ground Floor
HPC Workflows
Information
Solvers of differential equations often require long runs, making them unsuited for time-constrained tasks. Surrogate models are trained to approximate the outputs of these solvers, lowering computational costs.
Kitware partnered with EDF R&D to develop a proof of concept using the in situ capabilities of the ParaView application via the Catalyst library in order to visualize, track, and control the training of a deep learning surrogate model in real time.
Current monitoring tools lack the ability to display meaningful data in cases where standard numerical metrics are too shallow to reflect the nuances of the learning process. The proposed workflow leverages the scientific visualization capabilities of ParaView to better understand the training process.
Using a fluid dynamics problem, the Karman Vortex Street, we can easily locate where the training succeeds and where it fails. The steering capabilities of Catalyst 2 push this workflow forward by allowing the user to influence the learning process in real time. We successfully prototyped real-time modification of the learning rate and obtained immediate feedback.
Contributors:
Kitware partnered with EDF R&D to develop a proof of concept using the in situ capabilities of the ParaView application via the Catalyst library in order to visualize, track, and control the training of a deep learning surrogate model in real time.
Current monitoring tools lack the ability to display meaningful data in cases where standard numerical metrics are too shallow to reflect the nuances of the learning process. The proposed workflow leverages the scientific visualization capabilities of ParaView to better understand the training process.
Using a fluid dynamics problem, the Karman Vortex Street, we can easily locate where the training succeeds and where it fails. The steering capabilities of Catalyst 2 push this workflow forward by allowing the user to influence the learning process in real time. We successfully prototyped real-time modification of the learning rate and obtained immediate feedback.
Contributors:
- François Mazen (Kitware Europe)
Format
On-site