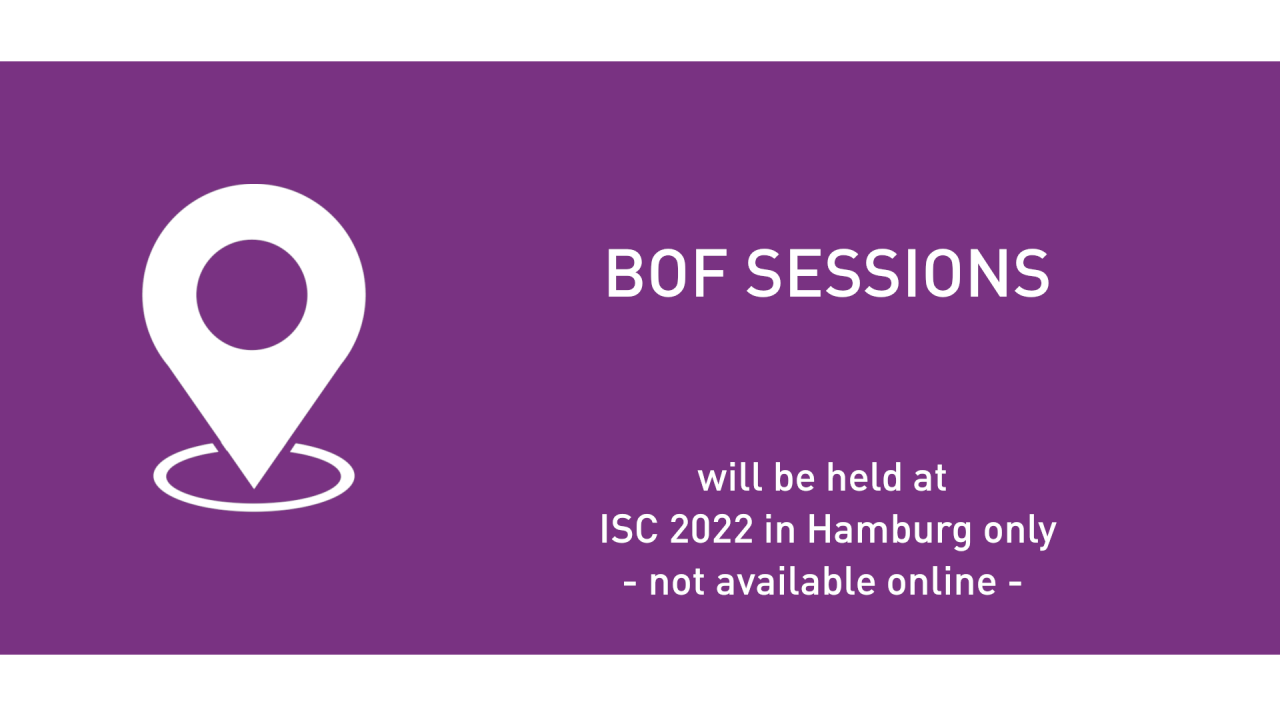
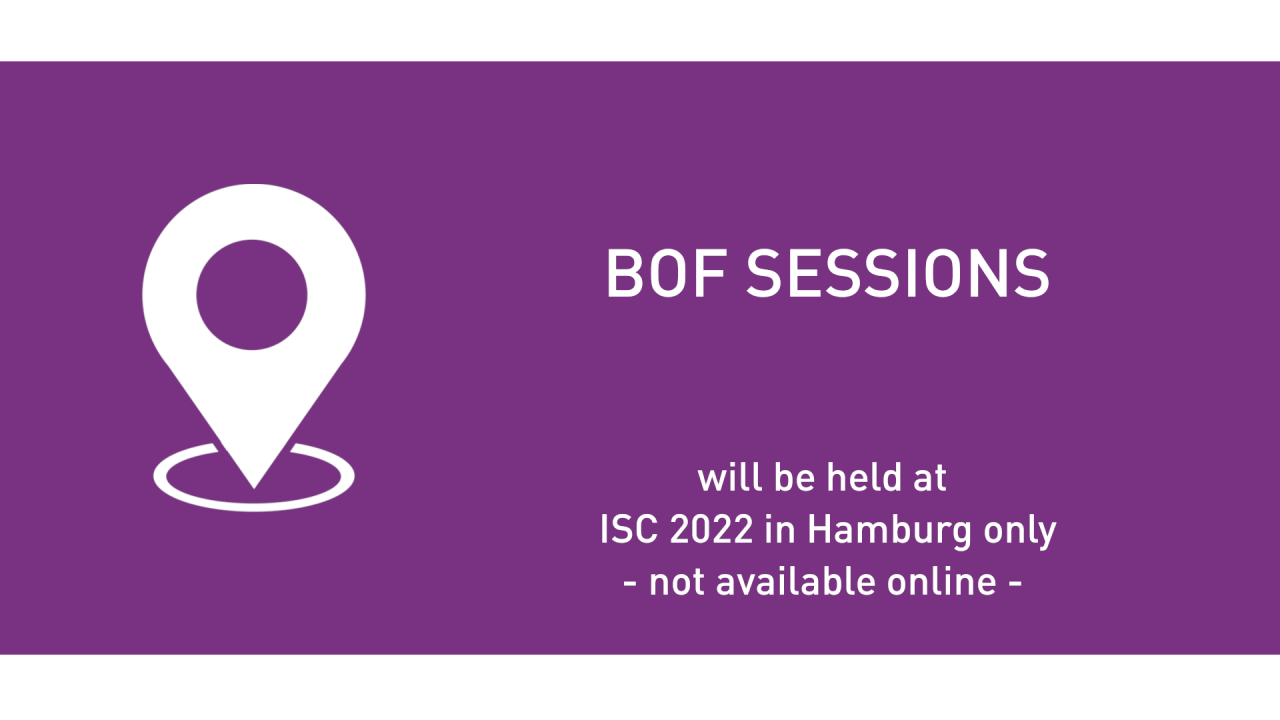
MLPerf: A Benchmark for Machine Learning
Wednesday, June 1, 2022 2:30 PM to 3:30 PM · 1 hr. (Europe/Berlin)
Hall E - 2nd Floor
Information
Machine learning applications are rapidly expanding into scientific domains and challenging the hallmarks of traditional HPC workloads.
Despite the breakneck pace of innovation, there is a crucial issue affecting the research and industry communities at large: how to enable fair and useful benchmarking of ML software frameworks, ML hardware accelerators, and ML systems. The ML field requires systematic benchmarking that is both representative of real-world use cases and useful for making fair comparisons across different software and hardware platforms. This is increasingly relevant as the scientific community is adopting ML in its research, such as model-driven simulations, analysis, surrogate models, and so on.
We present MLPerf, a community-driven system performance benchmark which spans a range of machine learning tasks. The speakers at this BoF are experts in the fields of HPC, scientific computing, machine learning, and computer architecture, representing academia, government research organizations, and private industry.
In this session, we will cover the past year’s development within the MLPerf organization and provide an update on v2.X of the MLPerf benchmark suite: we will discuss rule changes and additions and present recurring and newly added benchmarks. We are looking forward to discussing the state of MLPerf in general and MLPerf HPC specifically with the broader HPC community and solicit input for future developments of the benchmark suite.
Contributors:
We present MLPerf, a community-driven system performance benchmark which spans a range of machine learning tasks. The speakers at this BoF are experts in the fields of HPC, scientific computing, machine learning, and computer architecture, representing academia, government research organizations, and private industry.
In this session, we will cover the past year’s development within the MLPerf organization and provide an update on v2.X of the MLPerf benchmark suite: we will discuss rule changes and additions and present recurring and newly added benchmarks. We are looking forward to discussing the state of MLPerf in general and MLPerf HPC specifically with the broader HPC community and solicit input for future developments of the benchmark suite.
Contributors:
- Thorsten Kurth (NVIDIA Corporation)
- Peter Mattson (Google)
- Steven Farrell (Lawrence Berkeley National Lab/NERSC)
- Stefan Kesselheim (Forschungszentrum Jülich)
- Daniel Coquelin (KIT)
- Jeyan Thiyagalingam (Rutherford Appleton Laboratory, Science and Technology Facilities Council)
Format
On-site
Speakers
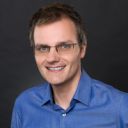
Thorsten Kurth
Senior Software EngineerNVIDIA Corporation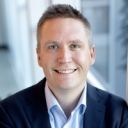
Peter Mattson
Senior Staff Engineer / PresidentGoogle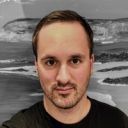
Steven Farrell
Machine Learning EngineerLawrence Berkeley National Laboratory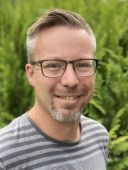
Stefan Kesselheim
Group LeaderForschungszentrum Jülich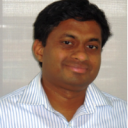
Jeyan Thiyagalingam
Senior ScientistRutherford Appleton Laboratory, Science and Technology Facilities Council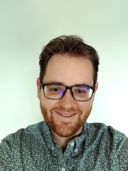
Daniel Coquelin
AI ConsultantKarlsruher Institut für Technologie (KIT)