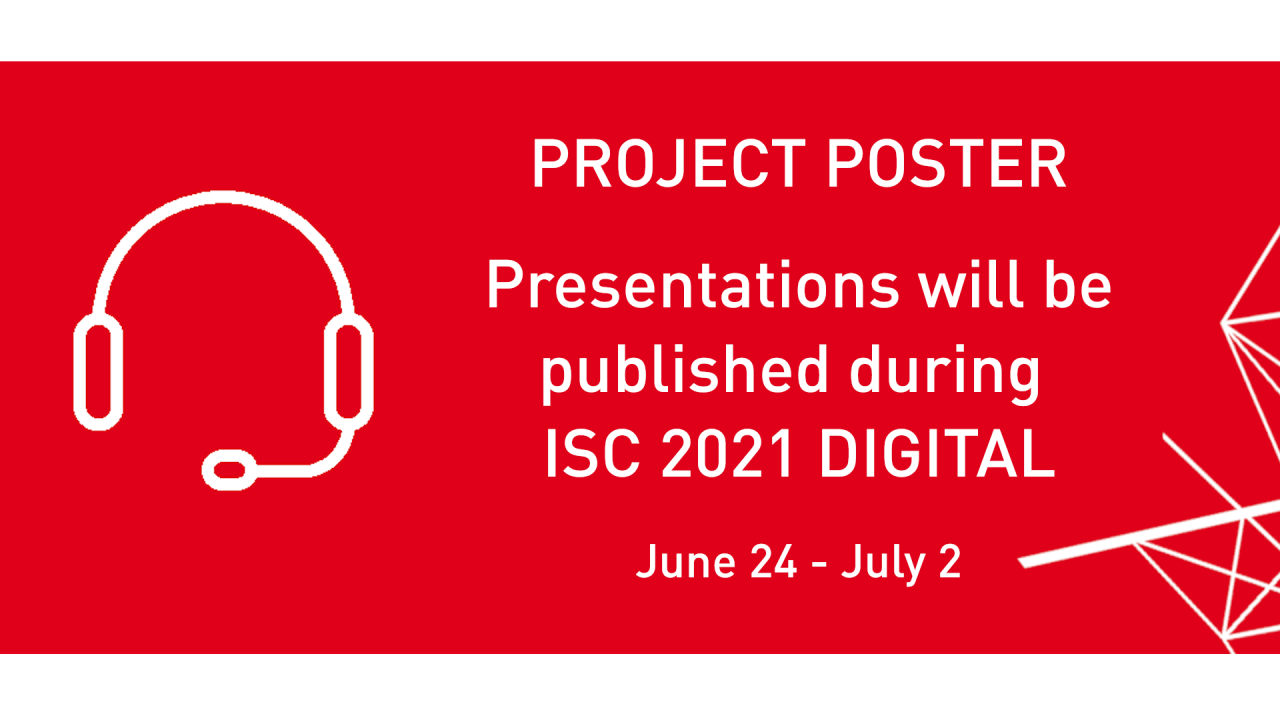
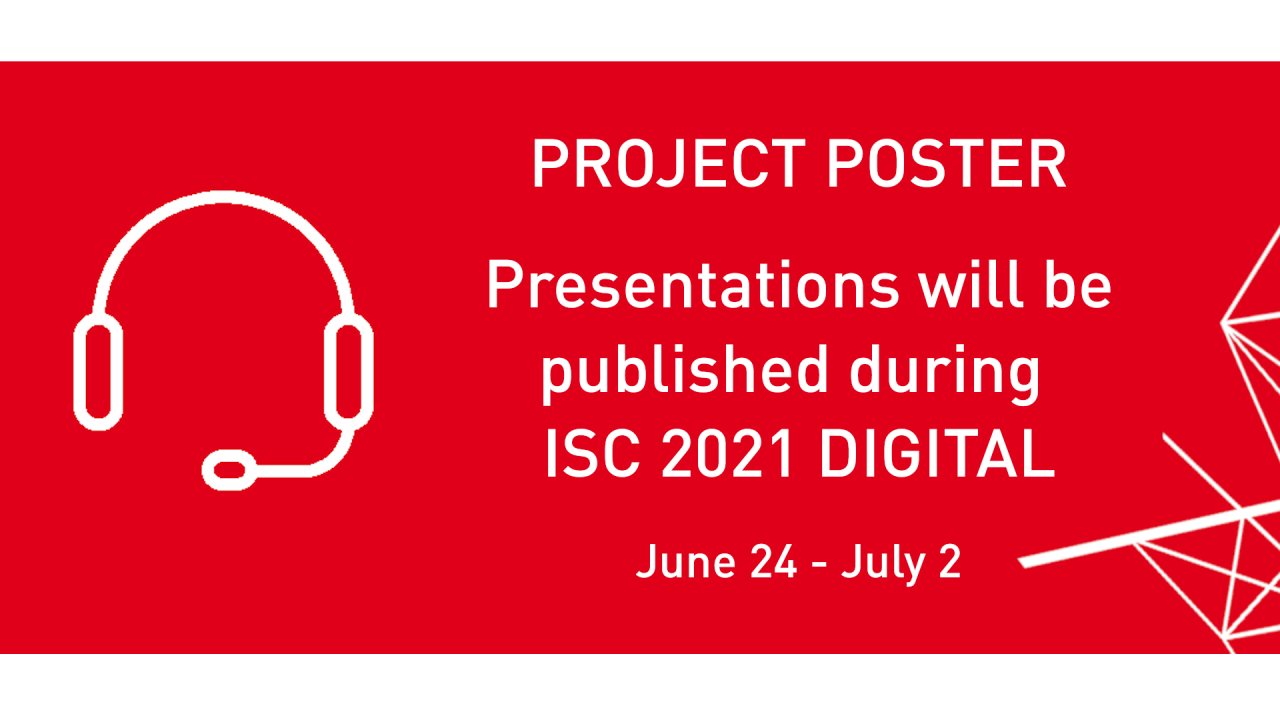
ExODE : Scaling the solving of Ordinary Differential Equation for Computational Biology
Only 126 seats left
Wednesday, June 30, 2021 3:00 PM to 4:00 PM · 1 hr. (Africa/Abidjan)
Exascale Systems
Information
Contributors:
Abstract:
In biology, the vast majority of systems can be modeled as ordinary differential equations (ODEs). Modeling more finely biological objects leads to increase the number of equations. Simulating ever larger systems also leads to increasing the number of equations. Therefore, we observe a large increase in the size of the ODE systems to be solved. A major lock is the limitation of ODE numerical resolution software (ODE solver) to a few thousand equations due to prohibitive calculation time. The AEx ExODE tackles this lock via 1) the introduction of new numerical methods that will take advantage of the mixed precision that mixes several floating number precisions within numerical methods, 2) the adaptation of these new methods for next generation highly hierarchical and heterogeneous computers composed of a large number of CPUs and GPUs. For the past year, a new approach to Deep Learning has been proposed to replace the Recurrent Neural Network (RNN) with ODE systems. The numerical and parallel methods of ExODE will be evaluated and adapted in this framework in order to improve the performance and accuracy of these new approaches.Visit the Project Website
Abstract:
In biology, the vast majority of systems can be modeled as ordinary differential equations (ODEs). Modeling more finely biological objects leads to increase the number of equations. Simulating ever larger systems also leads to increasing the number of equations. Therefore, we observe a large increase in the size of the ODE systems to be solved. A major lock is the limitation of ODE numerical resolution software (ODE solver) to a few thousand equations due to prohibitive calculation time. The AEx ExODE tackles this lock via 1) the introduction of new numerical methods that will take advantage of the mixed precision that mixes several floating number precisions within numerical methods, 2) the adaptation of these new methods for next generation highly hierarchical and heterogeneous computers composed of a large number of CPUs and GPUs. For the past year, a new approach to Deep Learning has been proposed to replace the Recurrent Neural Network (RNN) with ODE systems. The numerical and parallel methods of ExODE will be evaluated and adapted in this framework in order to improve the performance and accuracy of these new approaches.Visit the Project Website