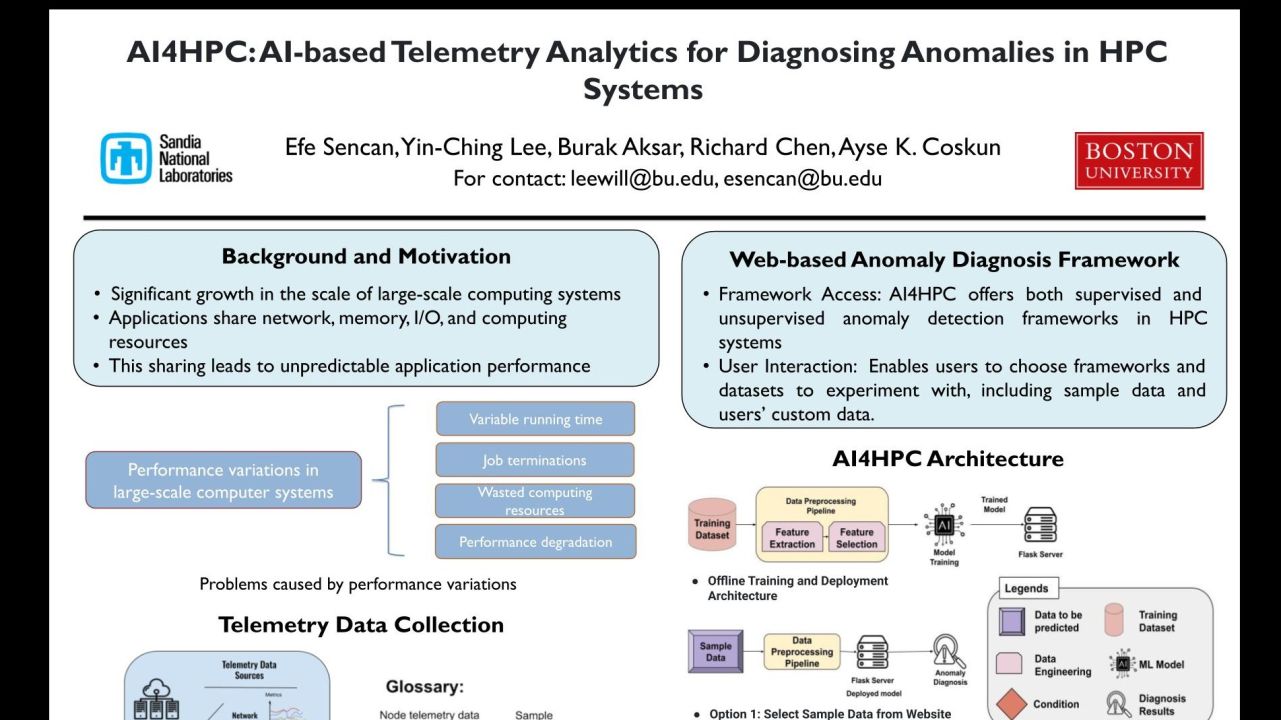
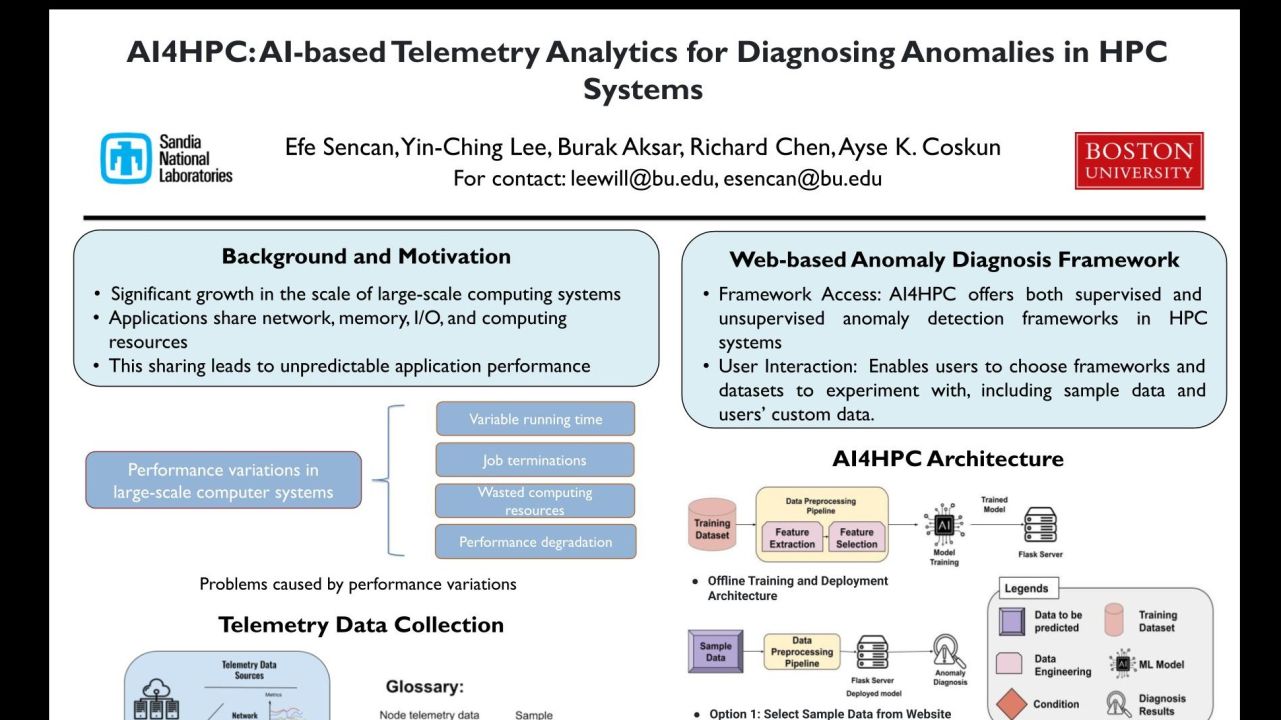
AI4HPC: AI-based Telemetry Analytics for Diagnosing Anomalies in HPC Systems
Monday, May 13, 2024 3:00 PM to Wednesday, May 15, 2024 4:00 PM · 2 days 1 hr. (Europe/Berlin)
Foyer D-G - 2nd floor
Project Poster
Community EngagementEngineeringHigh-Performance Data AnalyticsIndustrial Use Cases of HPC, ML and QCML Systems and Tools
Information
Poster is on display.
High-Performance Computing (HPC) systems are critical for scientific and societal advancements, capable of performing quintillion-scale calculations. These systems, however, face performance issues due to network contention, hardware malfunctions, and resource conflicts, affecting energy efficiency and increasing costs. HPC systems typically utilize advanced monitoring tools to track complex numeric multivariate time-series telemetry data, which is essential for resource usage analysis. The size and complexity of this data make manual analysis impractical, leading to the adoption of machine learning (ML) for automated performance analysis. To achieve practical and automated telemetry analysis, we developed ML-based frameworks suitable for different scenarios, including using supervised, semi-supervised, or unsupervised learning, tailored to the availability of “labeled” data. To enhance the accessibility and utility for the HPC community, we launched AI4HPC (https://ai4hpc.bu.edu/), a web platform dedicated to analysis with our ML-based performance diagnostics frameworks. AI4HPC offers easy access to models, enabling users to engage with our ML-based frameworks without having to install the frameworks on their systems. The platform features a user-friendly interface, designed to facilitate seamless interaction with our frameworks for users of varying technical backgrounds. Users can apply these frameworks to their own dataset or use sample datasets from the website, obtaining valuable insights through anomaly diagnosis and feature importance assessments. The web-based framework also enables users to provide targeted feedback on the anomaly diagnosis results for each of their application runs, playing a crucial role in the continuous improvement of ML-based frameworks.
Contributors:
High-Performance Computing (HPC) systems are critical for scientific and societal advancements, capable of performing quintillion-scale calculations. These systems, however, face performance issues due to network contention, hardware malfunctions, and resource conflicts, affecting energy efficiency and increasing costs. HPC systems typically utilize advanced monitoring tools to track complex numeric multivariate time-series telemetry data, which is essential for resource usage analysis. The size and complexity of this data make manual analysis impractical, leading to the adoption of machine learning (ML) for automated performance analysis. To achieve practical and automated telemetry analysis, we developed ML-based frameworks suitable for different scenarios, including using supervised, semi-supervised, or unsupervised learning, tailored to the availability of “labeled” data. To enhance the accessibility and utility for the HPC community, we launched AI4HPC (https://ai4hpc.bu.edu/), a web platform dedicated to analysis with our ML-based performance diagnostics frameworks. AI4HPC offers easy access to models, enabling users to engage with our ML-based frameworks without having to install the frameworks on their systems. The platform features a user-friendly interface, designed to facilitate seamless interaction with our frameworks for users of varying technical backgrounds. Users can apply these frameworks to their own dataset or use sample datasets from the website, obtaining valuable insights through anomaly diagnosis and feature importance assessments. The web-based framework also enables users to provide targeted feedback on the anomaly diagnosis results for each of their application runs, playing a crucial role in the continuous improvement of ML-based frameworks.
Contributors:
Format
On-site