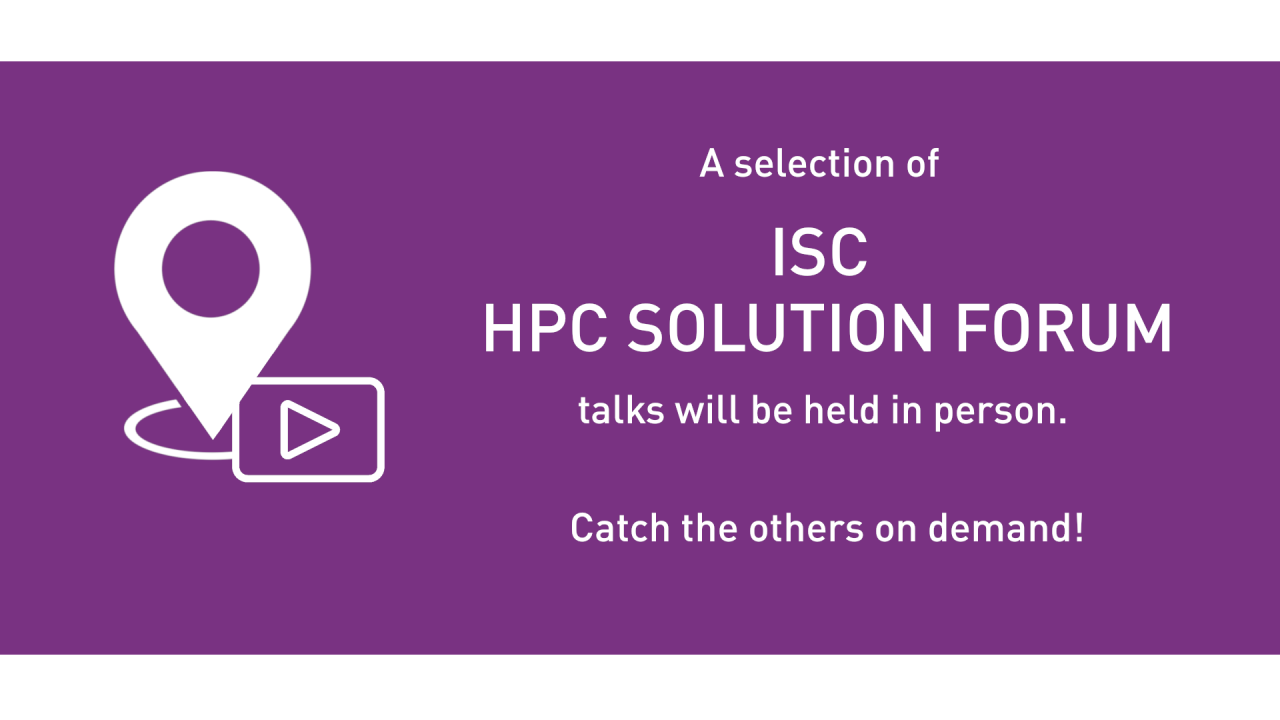
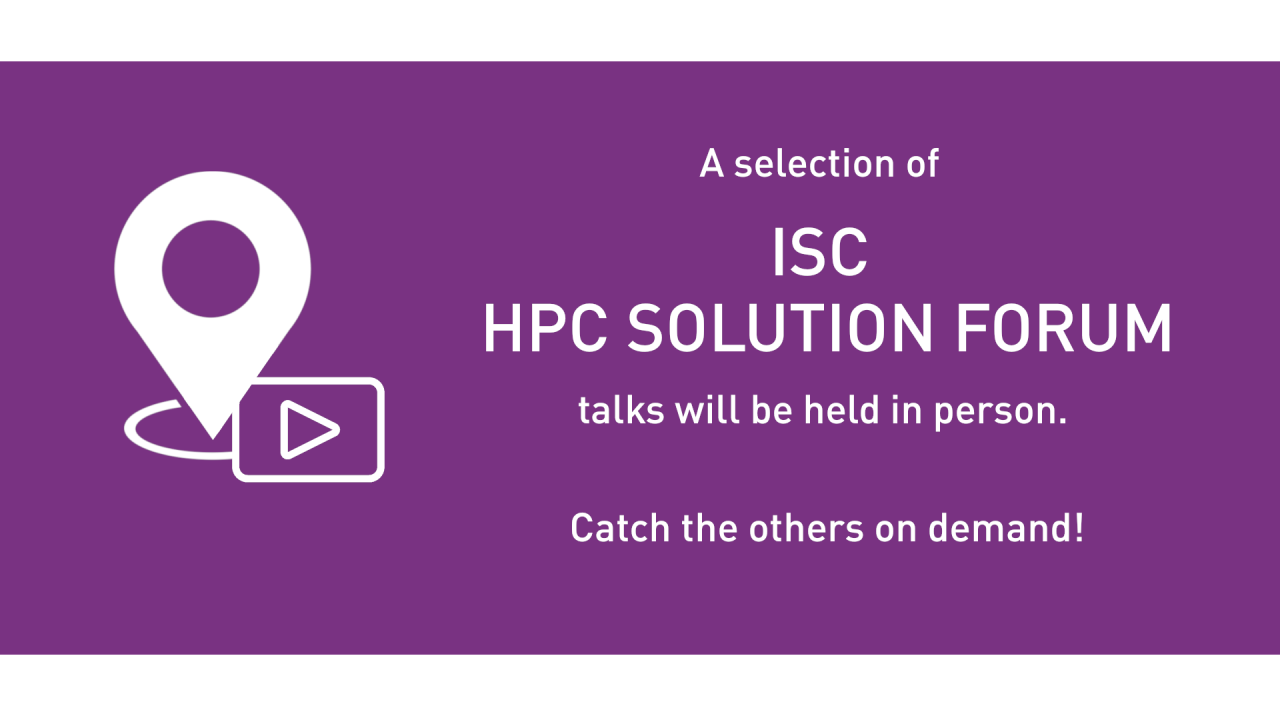
AI for Simulation: Demystified
Tuesday, May 31, 2022 1:00 PM to 1:20 PM · 20 min. (Europe/Berlin)
Hall H, Booth J901 - Ground Floor
Information
This presentation addresses the following topic(s):
However, due to the immense complexity of the calculations involved in many of these applications, researchers are often waiting a long time for simulation results to arrive. Speeding up these workflows by simply running the same programs on more powerful hardware can be very expensive, with a large cost often giving only a modest improvement in performance.
Clearly, a new approach is required to efficiently speed up these workloads, and many researchers are turning to machine learning models.
Graphcore’s Bow Pod systems, powered by the Intelligence Processing Unit (IPU), have been designed from the ground up to accelerate machine learning workloads, with plug-and-play connectivity for efficient datacentre scalability. The Bow Pod's disaggregated architecture means the CPUs reside separately from the IPUs, meaning the CPU:IPU ratio can be varied depending on the workload requirements. Bow Pod’s flexibility and performance enable scientists and researchers to accelerate HPC workloads using AI surrogate models without hindering the parts of their workloads which may rely on more traditional CPU-workload HPC techniques.
In this session, Graphcore’s Alex Titterton will explore a variety of scientific applications and use cases that use AI to speed up parts of their HPC workload.
- Machine learning and HPC: Each worthy of its own investment, or better together? (Alternately: Marriage for the ages, or heading for divorce?)
However, due to the immense complexity of the calculations involved in many of these applications, researchers are often waiting a long time for simulation results to arrive. Speeding up these workflows by simply running the same programs on more powerful hardware can be very expensive, with a large cost often giving only a modest improvement in performance.
Clearly, a new approach is required to efficiently speed up these workloads, and many researchers are turning to machine learning models.
Graphcore’s Bow Pod systems, powered by the Intelligence Processing Unit (IPU), have been designed from the ground up to accelerate machine learning workloads, with plug-and-play connectivity for efficient datacentre scalability. The Bow Pod's disaggregated architecture means the CPUs reside separately from the IPUs, meaning the CPU:IPU ratio can be varied depending on the workload requirements. Bow Pod’s flexibility and performance enable scientists and researchers to accelerate HPC workloads using AI surrogate models without hindering the parts of their workloads which may rely on more traditional CPU-workload HPC techniques.
In this session, Graphcore’s Alex Titterton will explore a variety of scientific applications and use cases that use AI to speed up parts of their HPC workload.
Format
On-site